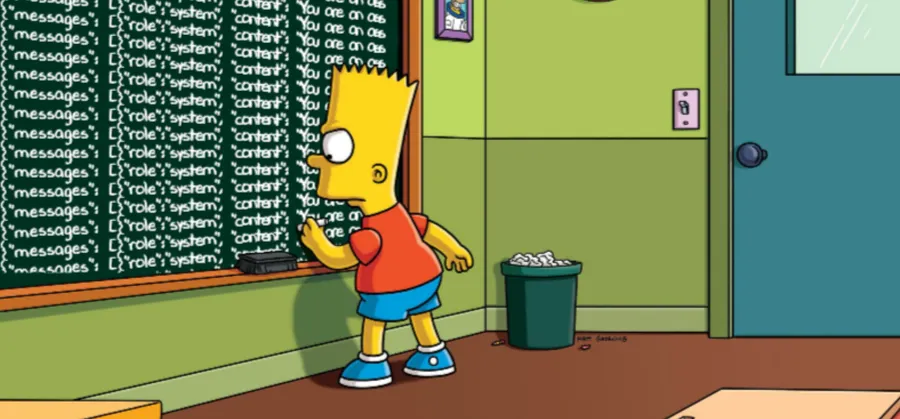
An Introduction to OpenAI fine-tuning

We’ve been tinkering with LLMs to write code on Val Town. Our platform runs vals - little snippets of TypeScript - with zero configuration, no deploying needed. These self-contained bits of functionality seem like the perfect target for something like GPT, with a little training. But we will need to teach GPT a little bit about the platform, which functions are available, how to structure its code.
OpenAI released fine-tuning for GPT-3.5 Turbo a couple of days ago: a way to teach your AI about how a problem area ahead of time, and save time and money on successive chat exchanges.
Is it worth it? Is it cheaper and better than regular prompt engineering? Let’s see.
What is fine-tuning?
Fine-tuning is a few-shot learning optimization. Few-shot learning is a prompt engineering technique where you provide examples of how to perform a task.
You are an assistant that misspells all words
User: Do you like music?Assistant: Yess, Iy luv musik a lott.
Fine-tuning lets you scale this approach:
- It let’s you pre-train on more examples than could fit in a prompt
- It reduces latency — no need to send over the same large prompt with every request
- It’s cheaper than GPT-4
When should you fine-tune?
You need fine tuning when:
- You have a lot of examples in your prompt
- The model will be run often, and you want it to run fast
- The AI agent needs to follow specific rules that require training
So, you probably don’t need fine-tuning at first. Fine-tuning is an optimization over a large number of examples in your prompt. If you don’t already have a prompt with many examples, start there. You should consider fine-tuning when you have so many that your prompt has become a burden. OpenAI recommends fine-tuning on at least 50 examples to see clear improvements. Fine tuning essentially front-loads the cost and time it takes to train a model, making future API calls faster.
It’s also important to try other prompt engineering techniques, prompt chaining, and function calling before jumping into fine-tuning. Bookmark this tutorial and come back to it when you need to further optimize your prompt.
How much does fine-tuning cost?
It depends what you compare it to! The following prices are per 100k tokens.
Fine-tuning | Input | Output | |
---|---|---|---|
gpt-3.5-turbo 4k | n/a | $0.15 (x1) | $0.2 (x1) |
gpt-3.5-turbo 16 | n/a | $0.3 (x2) | $0.4 (x2) |
gpt-3.5-turbo fine-tuned | $0.80 | $1.2 (x8) | $1.6 (x8) |
gpt-4 8k | n/a | $3 (x20) | $6 (x30) |
gpt-4 32k | n/a | $6 (x40) | $12 (x60) |
Fine-tuned Turbo is 8x more expensive than stock Turbo, but 2.5-7.5x cheaper than GPT-4. If you’re using stock Turbo, you’re likely looking at a price increase but performance increase. If you’re using GPT-4, fine-tuning can get you better pricing and response times. A common technique is to fine-tune Turbo on examples generated by GPT-4.
Getting started
If we haven’t scared you off and you’re ready to get started fine-tuning, the rest of this article will guide you through fine-tuning on a tiny data set. You can fork and run these examples by signing up for Val Town and putting your OpenAI token into your Val Town secrets.
1. Collect your examples
This dataset teaches our AI assistant to misspell every word in its reply. Each example is an object with one property, messages
, which contains an array of messages from either the system
, user
, or assistant
. The data itself is in the JSONL format.
2. Run a fine-tuning job
There are two steps to running a fine-tuning job: (1) uploading the data file, (2) starting the job. I created a couple helper function for this: one for uploading the file, one for starting the job, and one which combines these two steps into a single function. Below you can see how I use my helper functions, and the resulting fine-tuning job object:
3. Wait for the model to finish fine-tuning
About 10 minutes later, I got an email from OpenAI that my model was ready to use. The email also includes the model’s name, which you need to use it via the API.
4. Try out your model
I tested the fine-tuned model against stock Turbo with a prompt of just “misspell all words”
and no examples. In the example below, you can see stock turbo misspelled 4 out of 12 words vs the fine-tuned model misspelled 5 out of 9 words, which 20% more words misspelled. It’s also interesting to note that stock Turbo returned much lengthier replies unless I supplied a max_token parameter, while the fine-tuned model always returned short answers, just like the provided in the examples. Fine-tuning is a great way to train a model on style and tone.
Even with just this silly little example, we can see glimmers of the power of fine-tuning.
We at Val Town think there’s likely a lot more performance to be gained from simpler prompt engineering techniques, so we’re putting a pin fine-tuning for now.
If you end up trying it out, we’d be curious to hear how it goes for you. Come join us in the Val Town Discord!
Edit this page